We are proud to make something very complex into something understandable. Access to relevant, understandable, and actionable information is hard, and the more data there is, the harder it gets. To compound to this challenge, many of the traditional condition monitoring- based KPI’s like peak-to-peak and kurtosis are excellent metrics, however, these are understandable for specialized professionals like vibration analysts, yet not clear for others. To extend high-end condition monitoring to a larger fleet of assets, to bring the specialized technical vibration tools to the wider audience of maintenance professionals in an understandable and actionable way, we developed Coe, Condence Co-engineer, a new feature in the condence.io toolkit.
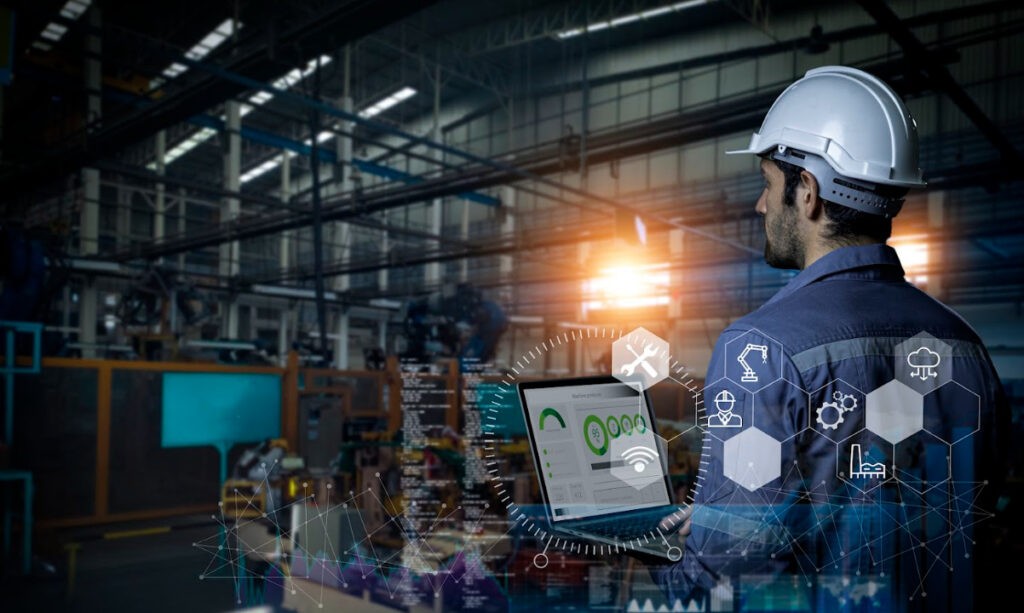
Coe is designed to make the job of maintenance professionals easier and more efficient. It builds on multiple Condence capabilities. It helps automate the analysis of vibration data, enabling users to detect potential issues and anomalies that may not be detectable through traditional threshold monitoring and gives more lead time to plan and address the budding issues.
By using Coe, users can save time and reduce costs associated with equipment repairs and downtime. Coe’s ability to learn and adapt over time means that maintenance professionals can improve their equipment maintenance strategies, enabling them to make more informed decisions about maintenance tasks and scheduling.
How Coe Works
Coe is a powerful machine learning tool that analyses vibration data from sensors installed on industrial equipment. It uses special methods to analyze data and convert it to understandable metrics that indicate e.g. bearing condition, lubrication, or mechanical health. This will give the operator an understandable KPI to follow.
Sometimes access to kinematic information can be a challenge or take longer to access, especially when dealing with older machines. Coe does not require kinematic information or even RPM information to create an analysis and reports.
The system automatically detects patterns or anomalies that indicate potential issues or failures and prompts the user to act, either by suggesting a specific maintenance or repair task. The system also adapts and learns over time, becoming more accurate and effective as it receives more data and feedback.
Real-World Examples
In the development phase, Coe was put into real-life tests. Tens of actual failures and cases from various industries have been tested head-to-head. In all the cases, Coe detected the failure before the vibration specialist responsible for analyzing the machine. To demonstrate the power and benefits of Coe, we have two real-world examples that showcase the capabilities of this feature:
Case Study 1: Industrial Fan
An industrial fan in a manufacturing plant had been experiencing issues with their equipment, specifically with bearing failures both in electric motors and fans. This had resulted in costly repairs and downtime. The company had implemented a vibration-based condition monitoring program with traditional threshold monitoring to detect issues in their equipment.
The company decided to implement Coe, and the system detected an emerging bearing failure over a year before traditional threshold monitoring would have alerted the user to the issue. The system provided actionable recommendations, enabling the company to perform maintenance before the equipment failed, saving them money, and avoiding downtime.
Case Study 2: Industrial Gearbox
A user of industrial gearboxes manually monitored their equipment to detect issues. However, this process was time-consuming, hard to scale and costly, and they were looking for a more efficient and effective solution.
Coe detected the first indications of an emerging gear wheel issue approximately a year before the user deemed that further actions were required. Coe provided recommendations for maintenance actions well in advance, enabling the company to address the issue before it led to equipment failure and downtime.
Conclusion
Condence Co-engineer is a powerful tool that allows our customers to monitor their equipment effectively and efficiently. By harnessing the power of machine learning, Coe can detect potential issues and anomalies that traditional threshold monitoring cannot. With Coe, customers can reduce downtime, save money on repairs, and increase their overall operational efficiency.
Implementation of Coe on existing installations or installations in progress can be done as a global or asset specific update that does not require any changes in the field.
Coe will be available for the Advisory group in May. The commercial release date is scheduled for the end of June. If you are interested in joining the Advisory group or have a case you would like to test Coe on, please contact us.
0 Comments