Downtime is expensive. Effective monitoring solutions for industrial maintenance can reduce downtime and give your company a competitive advantage in managing your assets over the long term.
However, understanding what artificial intelligence (AI) and machine learning concepts mean to an organisation can be a challenge.
Many opinions and expectations exist around AI and machine learning techniques. But are they buzzwords or a practical solution?
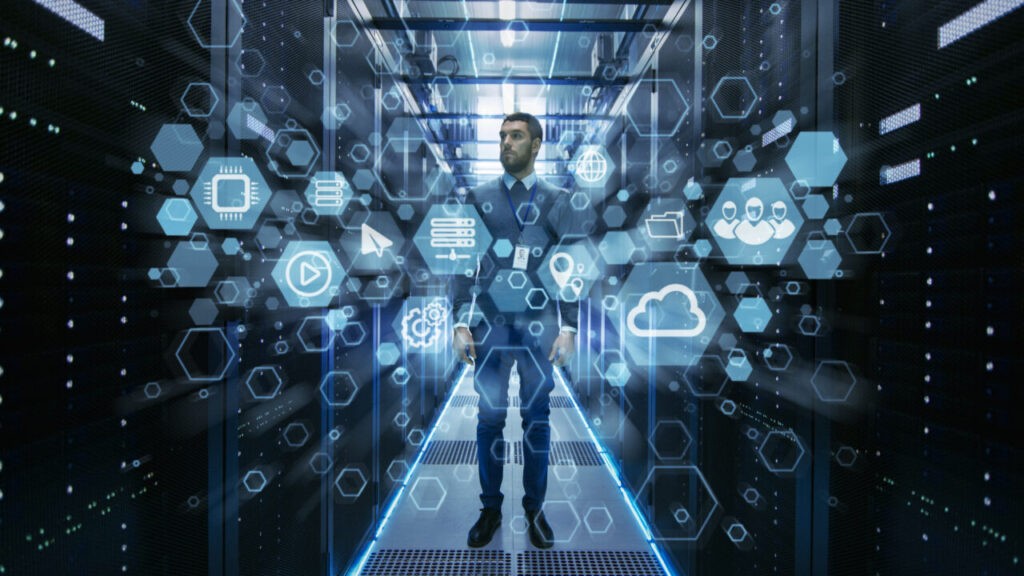
In reality, these tools offer a nuanced approach that can elevate how companies manage their industrial maintenance workload. AI and machine learning are powerful tools, and they should be used as such rather than as a blanket answer to all problems when developing effective maintenance solutions.
In this blog post discussing AI and its role in industrial maintenance, we’ll explore:
- Why opinions on AI and machine learning are so divided
- The growing potential of AI for industrial maintenance
- Building collaboration between humans and machines
- How using the power of AI can drive better business outcomes
Why are opinions so divided on AI and machine learning?
Some say companies can’t use AI and machine learning for condition monitoring and predictive maintenance because all industrial installations are different. There is simply not enough good data available, and because the digital journey begins with quality data, this can be a significant obstacle to widespread adoption.
Others think AI tools can solve a wide variety of issues in the industry, believing it must be quick and easy to simply pull whatever data you need from a machine at any time.
There is truth on both sides of the debate. Manufacturers have built far more reliability into industrial machines nowadays than in the past, according to this presentation from Reliability Web. The future of machine condition monitoring must consider how these assets are built and what we need to do to keep them from failure.
For example, companies don’t want to spend too little on monitoring and analysing asset condition, but ideally, they won’t spend too much either. Optimising expense versus impact should be your ultimate goal.
Condition monitoring solutions should help you focus on the data that matters to prescriptive maintenance and preventing machine failure. Tracking and analysing quality data is critical to helping you make better decisions regarding industrial maintenance.
A balanced view of what AI and machine learning can do for your company is key to data-driven decisions that save you money—and that you can scale within your organisation for the best return.
The growing potential of AI for industrial maintenance
AI has taken giant steps as a data science in recent years. Long ago, companies used only mathematical and statistical methods to group data and extract information from it. That process required a vast amount of special knowledge to build even simple models; still, the results were unstable.
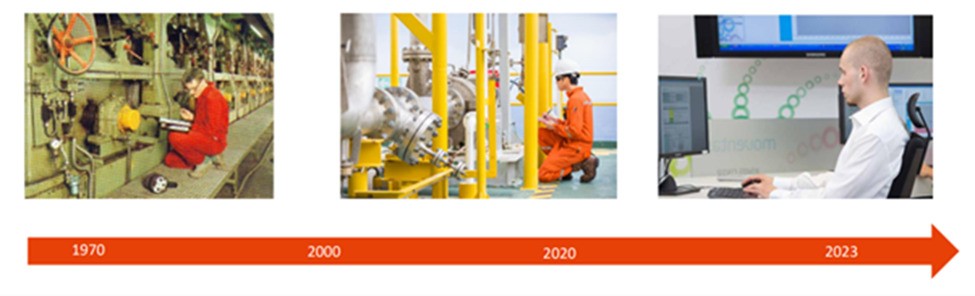
Then, the software industry found mathematical tools. They made libraries to help developers worldwide reduce the knowledge required to use complex algorithms and accelerate the development time to build complex products. Still, even with libraries available, it required a lot of custom craftsmanship from case to case, which was neither efficient nor reliable.
Now, you can find packaged products on the market which are easier to build reliably. A massive effort is already put into the products to fit different models and methods so we can intelligently pull the best results from the best-fitting algorithms.
Building collaboration between humans and machines
Machines do an excellent job of automating data collection at a massive scale, all while consistently and thoroughly monitoring devices. However, real people must be part of the process to review the findings and determine if the recommendations made from the data are appropriate, as well as to make adjustments if necessary.
Machines can see anomalies that humans can’t and can provide cross-company analyses for a broader view of optimal maintenance scheduling. Humans need to make decisions that drive company growth and profitability.
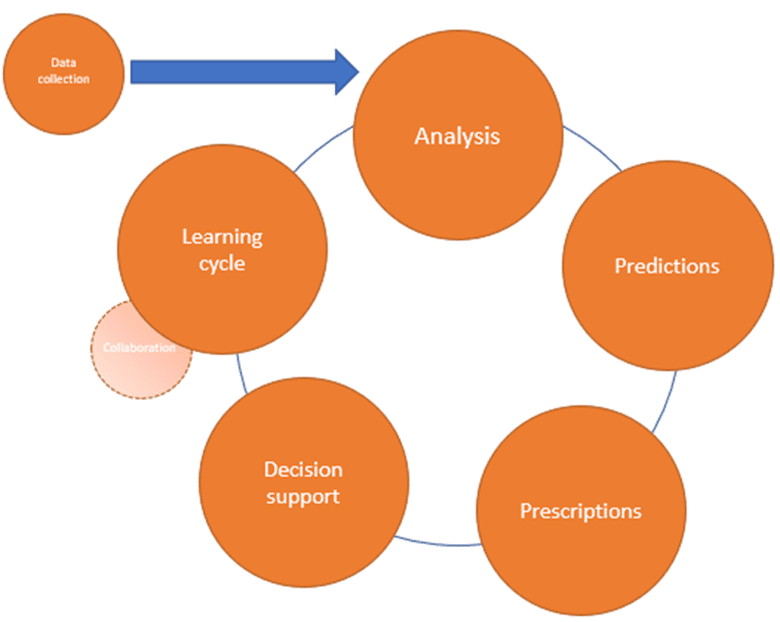
The best solution is a balanced approach, where you tap into the strengths of human resources and machines and scale your capabilities from there.
AI product manufacturers often demonstrate these devices with tasks related to forecasting failures on production lines only hours (or minutes) in advance. Unfortunately, that’s not enough for most industrial clients and still leaves the risk of machine failure or expensive repairs. You need valuable information to schedule repairs and maintenance well in advance to maximise productivity and uptime. This approach makes a measurable impact on costs and risk reduction.
To benefit from AI and machine learning, look for deeper insights, such as the optimal timing for necessary maintenance. This kind of data and analysis creates robust, prescriptive maintenance recommendations. You can combine well-organised, traditionally collected and analysed data with modern AI and machine learning tools to scale how you manage your industrial assets over the long term.
How using the power of AI can drive better business outcomes
Preventing failures of critical equipment to avoid unnecessary downtime is vital to effective industrial maintenance. Machines allow humans to scale monitoring, data collection and analysis, and provide the opportunity for insightful, data-driven decision-making.
As companies shift to a prescriptive maintenance model over a more traditional response, there is deep value in reorienting the roles of humans and machines. Humans are great logical thinkers and decision-makers. Devices can tirelessly harvest information from the masses of assets and simplify evidence and insight for that decision-making.
When human resources and machines are used wisely to drive better business outcomes, companies save time and money and lower their risk of loss.
AI and machine learning aren’t instant solutions for asset management, but they are powerful tools and assistants in the right hands. They can significantly impact reliability and collateral losses, especially when we remove the unexpected element from the equation in collaboration with humans and machines.
Our solution for deep collaboration between machines and humans is a Digital Engineer that operates as an assistant to those responsible for the well-being of industrial assets. The Digital Engineer changes the bias of traditional asset management and automates routine measurements that once were executed by humans.
Exploring how machines and humans can collaborate for a powerful shift in industrial asset management can be vital to your company’s operations. Contact us to book a demo.
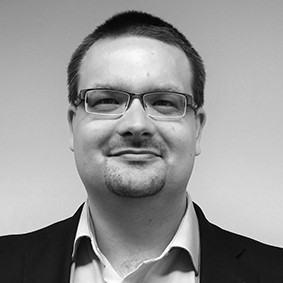
Pasi Lahti / CTO
pasi.lahti@distence.fi@distence.fi
0 Comments